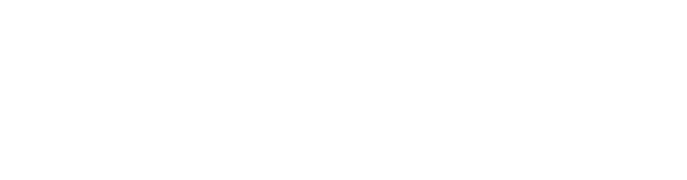
Gene expression profiles contribute to robustly predicting prognosis in hepatocellular carcinoma
Hepatocellular carcinoma (HCC) is characterized by both inter-and intra-tumor heterogeneity and has distinct clinical outcomes. A promising clinical tool to perform patient stratification, prognosis evaluation, and treatment recommendations is indispensable. Here, we enrolled a total of 1595tumorpatientsfrom13independentcohorts, including seven cohorts with survival data, four cohorts with immunotherapy information, and two cohorts with transcatheter arterial chemoembolization (TACE) and Sorafenib information, respectively (Table S1). Using 96 algorithms combinations derived from 10 popular machinelearning approaches, a novel framework was constructed and described in Figure S1. Firstly, a total of 26 stable consensus prognostic genes were screened in seven cohorts harboring complete survival information via univariate Cox regression analysis. Then, these 26 genes were further subjected to our integrative machine learning-based framework to establish a consensus prognostic signature (CPIS). For a prognostic signature, superior generalization capability is a target leading research to be pursued, which means the signature still retains robust performance across different validation cohorts. Hence, the C-index was measured in the other six validation cohorts and the signature with the highest average C-index (0.682) was regarded as the optimal one, which was derived by the classical machine learning algorithm Ridge.